About this event
If you are unable to join in person for this symposium, please join for the livestream on the CCAI YouTube channel: www.youtube.com/@ClimateChangeAI
Join us at the forefront of innovation and environmental stewardship at the University of California, Berkeley for a pivotal symposium on March 26-27, 2024. This gathering will convene experts across the fields of artificial intelligence and the natural sciences to explore groundbreaking interventions in climate change technology.
This symposium will have two foci:
- Accelerating Discovery: Development of AI and techniques to accelerate or otherwise revolutionize the discovery process and timeline.
- Pathways to Deployment: Chart the course for deploying emergent technologies that promise significant strides in society's ability to adapt to and mitigate the effects of climate change.
Engage with an exclusive academic audience, limited to 150 participants, ensuring a focused and intensive exchange of ideas. This is an unmissable opportunity for those committed to shaping a sustainable future through scientific advancement and technological innovation.
Event Schedule
- Omar Yaghi
Digital Reticular Chemistry - Kristin Persson
Data-Driven Understanding of Materials and Molecular Reactivity - Austin Sendek
Leveraging AI to develop new materials for global decarbonization
AI-for-climate: A call for application-driven innovation
|
- Connor Coley
Accelerated molecular design, synthesis, and analysis - Aditi Krishnapriyan
Machine learning methods for improving molecular simulations - Larry Zitnick
Modeling Atoms to Address Our Climate Crisis - Carla Gomes
AI for Scientific Discovery and a Sustainable Future
- Soukayna Mouatadid
Machine Learning for Improved Sub-Seasonal Forecasting - William Collins
Studies of Extreme Weather using Machine Learning and Climate Emulators - Aditya Grover
Foundation Models for a Sustainable Planet
Featured Speakers

Omar Yaghi
Digital Reticular Chemistry
Our recent work on digitalization of reticular chemistry will be reported. We have been experimenting with ChatGPT and machine learning in the discovery of new MOFs for clean air, water, and energy applications.
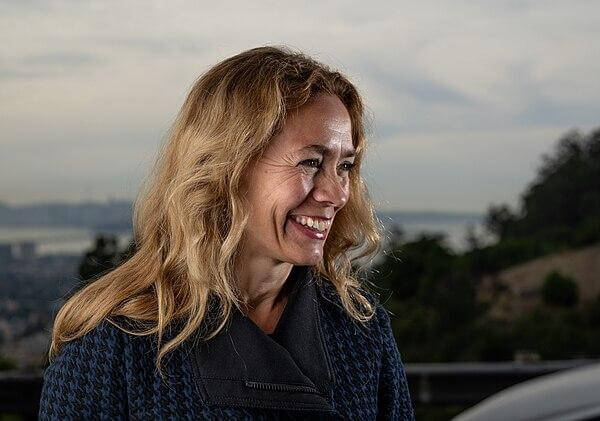
Kristin Persson
Data-Driven Understanding of Materials and Molecular Reactivity
Computational screening of materials for specific functional properties is rapidly changing the landscape of materials and molecular design. However, computational evaluation and guidance for synthesizability and reactivity as a function of the environment remain challenging due to the complexity of the available phase space. Here we present data-driven first-principles frameworks using a combination of high-throughput calculations, reaction networks, and machine learning to i) predict decomposition of liquid electrolytes and ii) guide solid state synthesis. Our automated methodology for capturing the electrode-electrolyte reactivity is based on a systematic generation of relevant species using a general fragmentation/recombination procedure which provides the basis for a vast thermodynamic reaction landscape, calculated with density functional theory. We explore this landscape using stochastic methods and shortest pathfinding algorithms, which yield the most likely reaction pathways which are then refined with transition state calculations and kinetic information. The results of the framework show promise in being able to automatically recover previous insights on single reaction pathways, as well as successfully predicting the early dynamics and competitive nature of the solid-electrolyte interface formation. In the case of solid-state synthesis, we leverage the Materials Project (www.materialsproject.org) thermodynamic phase diagrams to construct a reaction network model that mimics the sequential nature of solid-state reactions for stoichiometric compounds. We track the overall Gibbs free energy as a function of precursor selection and propose two selectivity metrics, primary and secondary competition, to assess the favorability of target/impurity phase formation in solid-state reactions. These metrics are evaluated against literature reactions and tested against nine proposed precursor selections; both traditional and non-traditional; for the synthesis of BaTiO3. Finally, we give our perspective on the era of data-driven materials design; pitfalls and promises.
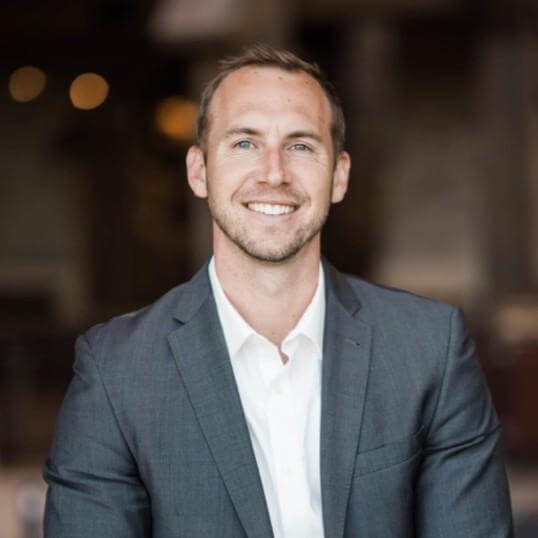
Austin Sendek
Leveraging AI to develop new materials for global decarbonization
Materials science is undergoing a massive paradigm shift, driven by dual revolutions: exponential increases in computing power, and power-law increases in the accuracy of A.I. models. In order to help us meet our net zero climate goals by 2050, Aionics is leading the charge to use these rapidly-improving computational methods to accelerate the materials discovery and commercialization process. In this talk, Cofounder and CEO Dr. Austin Sendek will discuss how materials science is changing and what to expect for the future.
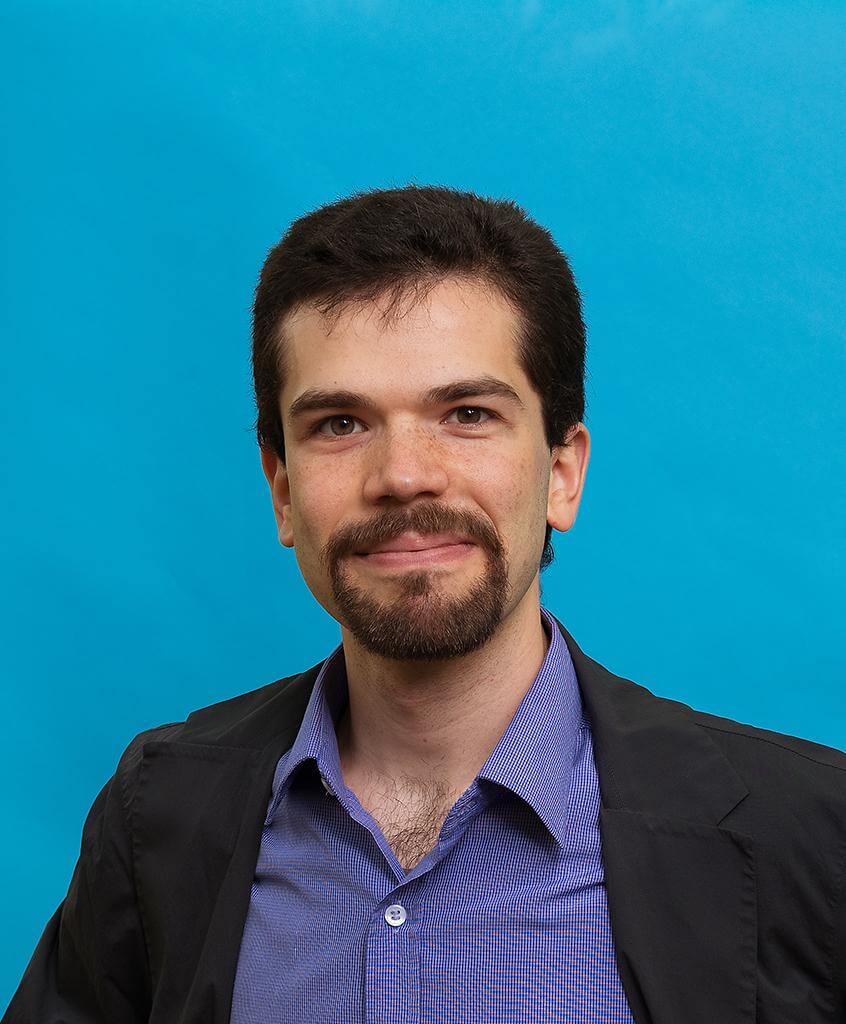
David Rolnick
AI-for-climate: A call for application-driven innovation
Machine learning is increasingly being used to help tackle climate change, from accelerating climate models to improving materials for batteries. As such applications grow, however, it is becoming clear that standard ML tools often fall short. High-powered algorithms designed using traditional benchmarks may fail to capture the constraints or metrics of specific real-world problems, while a “one size fits all” approach ignores useful auxiliary information in specific applications. In this talk, we show how application-driven innovation can lead to ML algorithms that are both methodologically innovative and highly impactful in the fight against climate change.
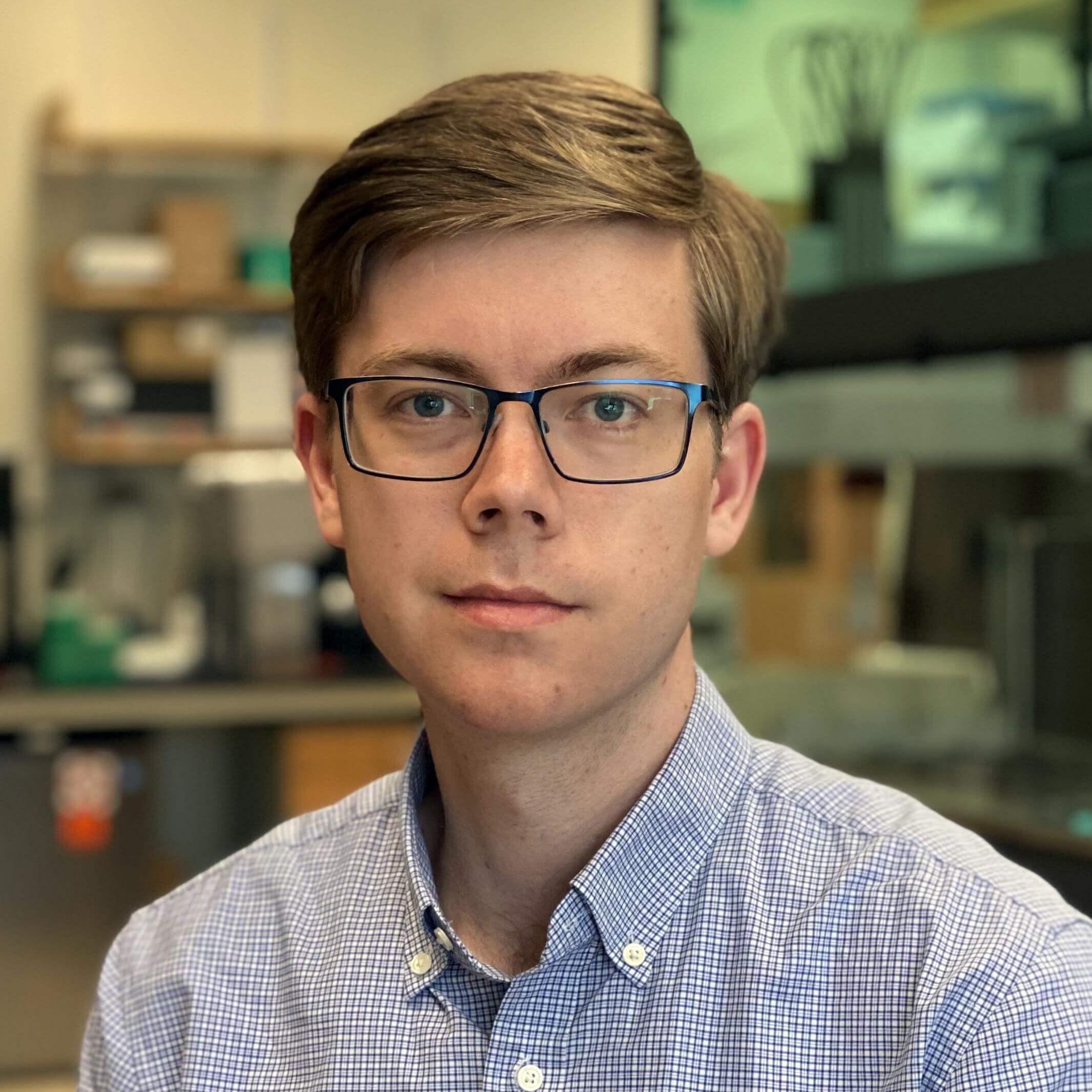
Connor Coley
Accelerated molecular design, synthesis, and analysis
Artificial intelligence and machine learning have become important components of the computational toolbox that can be used to advance chemical research and discovery. In this talk, I will provide an overview of the many areas where molecular discovery can be and has been accelerated by these techniques. Specifically, we will discuss about how surrogate structure-property models enable the exploration of massive virtual libraries of candidate materials, how generative modeling can be tailored to the reality of physical experimentation, how historical data on chemical reactions helps us recommend synthetic routes to access molecules of interest more sustainably, and how workflows in structure elucidation help us measure human and planetary health. These far-reaching topics highlight the many ways that AI for chemistry is evolving to help solve critical scientific challenges.
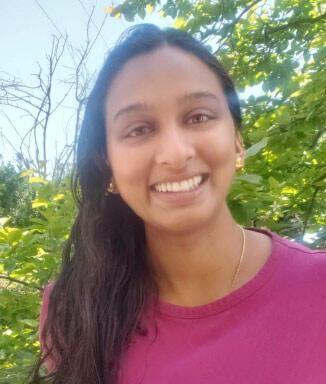
Aditi Krishnapriyan
Machine learning methods for improving molecular simulations
Molecular simulations aim to model the spatiotemporal behavior of atomistic systems throughout biology, chemistry, and materials science. Given the computational burden of running such simulations for long timescales, machine learning force fields, and particularly neural network interatomic potentials, are an attractive alternative to ab initio methods. This talk will focus on addressing current machine learning challenges in this space, with an emphasis on broad learning strategies that are applicable across a wide variety of systems and neural network architectures. I will discuss training procedures to improve the stability of molecular simulations over time, as well as formulations of symmetry operations in neural networks to learn more accurate representations of these atomistic systems. The applicability of these methods extends to other scientific domains, including neural PDE solvers. Finally, I will discuss how even as we build larger datasets in the future, these learning strategies remain general, as well as independent to specific neural network architectures.
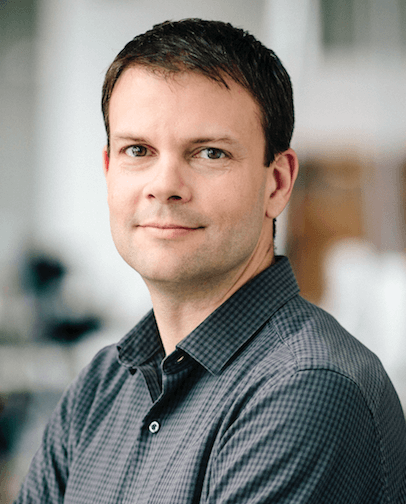
Larry Zitnick
Modeling Atoms to Address Our Climate Crisis
Climate change is a societal and political problem whose impact could be mitigated by technology. Underlying many of its technical challenges is a surprisingly simple yet challenging problem; modeling the interaction of atoms. In this talk, we motivate the problem and provide insights into how this opens up new intriguing directions for machine learning and AI researchers. Recent large-scale datasets released by the Open Catalyst Project enable the training of ML models that generalize across a broad range of the chemical space. We conclude by exploring the numerous open problems and their potential for wide ranging impact beyond climate change.
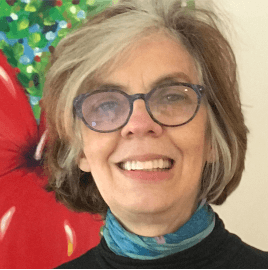
Carla Gomes
AI for Scientific Discovery and a Sustainable Future
Artificial Intelligence (AI) is a rapidly advancing field, achieving remarkable milestones ranging from computer vision and machine translation to world-champion level Go gameplay, self-driving cars, and Chat-GPT. The ever-growing AI capabilities unlock promising opportunities for progress in various domains. I will discuss our AI research devoted to fostering scientific discoveries for a sustainable future. Specifically, I will talk about our efforts in the new interdisciplinary field of Computational Sustainability, which aims to harness computational models and methods that enable a balance between environmental, economic, and societal needs. I will present examples of computational sustainability challenges, encompassing biodiversity conservation, strategic planning of hydropower dams in the Amazon basin, and the discovery of renewable energy materials. I will highlight cross-computational themes and challenges that AI poses, underscoring the potential for groundbreaking advancements in pursuing a sustainable future.
Animashree Anandkumar
AI Accelerating Scientific Understanding
While language models have impressive capabilities of text understanding, they lack physical understanding and grounding needed in scientific domains. For instance, language models may suggest new hypotheses such as new molecules or designs, but they lack physical validity and the ability to internally simulate the processes. Hence, the proposed hypotheses still require physical experimentation for validation which is the biggest bottleneck of scientific research. Numerical simulations offer an alternative for physical experiments, but traditional methods are too slow and infeasible for complex processes observed in many scientific domains. We propose AI-based simulation methods that are 4-5 orders of magnitude faster and cheaper than traditional simulations. They are based on Neural Operators which learn mappings between function spaces, and have been successfully applied to weather forecasting, fluid dynamics, carbon capture and storage modeling, and optimized design of medical devices, yielding significant speedups and improvements.
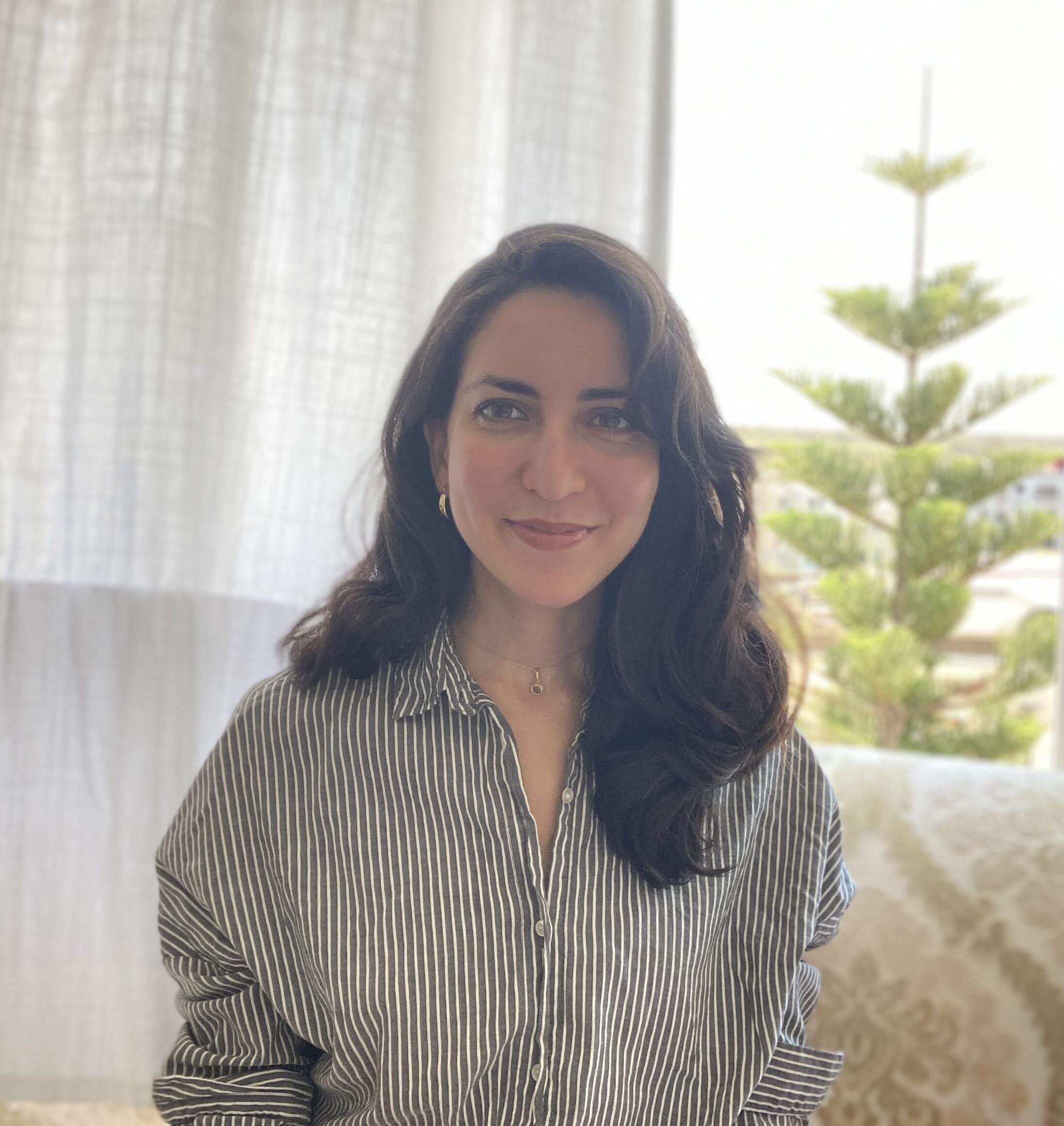
Soukayna Mouatadid
Machine Learning for Improved Sub-Seasonal Forecasting
Subseasonal forecasting—predicting temperature and precipitation 2 to 6 weeks ahead—is critical for effective water allocation, wildfire management, and drought and flood mitigation. Recent international research efforts have advanced the subseasonal capabilities of operational dynamical models, yet temperature and precipitation prediction skills remain poor, partly due to stubborn errors in representing atmospheric dynamics and physics inside dynamical models. In this talk, I will present my research towards leveraging machine learning to efficiently improve the accuracy of weather and climate predictions. First, I will illustrate how we can train neural networks to emulate the full dynamics of a numerical weather prediction model and predict the global atmospheric flow in the medium-range. Next, I will introduce a hybrid dynamical-learning approach that can outperform both numerical weather prediction models as well as state-of-the-art learning models on the subseasonal timescales. I will then discuss how we can couple these performance improvements with a practical workflow to explain the achieved skill gains and identify higher-skill windows of opportunity based on specific climate conditions. Finally, I will discuss future directions and challenges in data-driven weather and climate prediction.
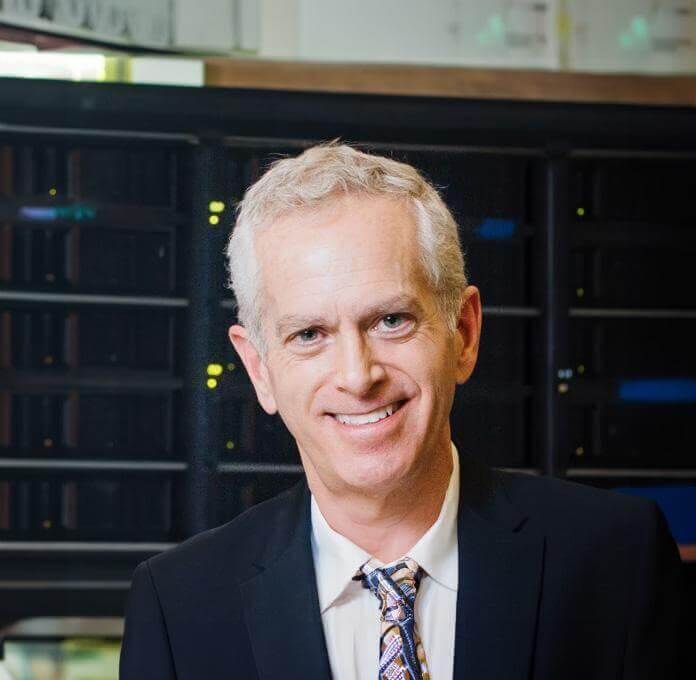
William Collins
Studies of Extreme Weather using Machine Learning and Climate Emulators
Studying low-likelihood high-impact climate events in a warming world requires massive ensembles of hindcasts to capture their statistics. It is currently not feasible to generate these ensembles using traditional weather or climate models, especially at sufficiently high spatial resolution. We describe how to bring the power of machine learning (ML) to generate climate hindcasts at four to five orders-of-magnitude lower computational cost than conventional numerical methods. We show how to evaluate ML climate emulators using the same rigorous metrics developed for operational numerical weather prediction. We conclude by discussing the prospects for studying the causes and statistics of low-likelihood high-impact extremes using huge ensembles generated using these ML emulators.
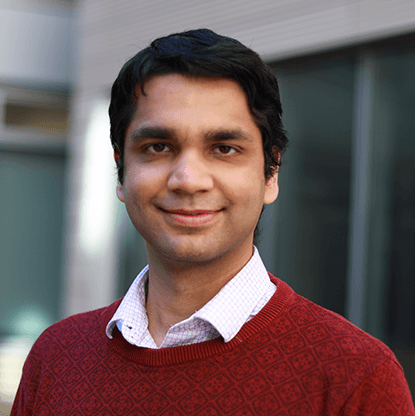
Aditya Grover
Foundation Models for a Sustainable Planet
Key global sustainability challenges, ranging from ensuring food and energy security to managing disaster response, critically depend on our ability to accurately forecast weather and project climate. While current approaches are limited by our physical understanding of the atmosphere, improvements in sensory capabilities and large-scale machine learning (ML) present immense opportunities for designing alternative solutions. In this talk, I will discuss key design principles for developing foundation models for atmospheric sciences. Unlike language and vision, scientific domains present unique challenges due to the significant heterogeneity of available datasets. Moreover, downstream tasks in atmospheric sciences require generalizing across a broad range of variables and spatiotemporal resolutions. To address these challenges, I will demonstrate novel strategies for data engineering, model design, spatiotemporal optimization, and cross-modal finetuning aimed at scalable learning of atmospheric foundation models. Our resulting models exhibit exceptional skill, speed, and adaptability across a range of predictive tasks in weather and climate science. Finally, I will summarize the broader implications of this research for scientific ML and growing external efforts to adapt our models for diverse sustainable development goals.
|
Moderators
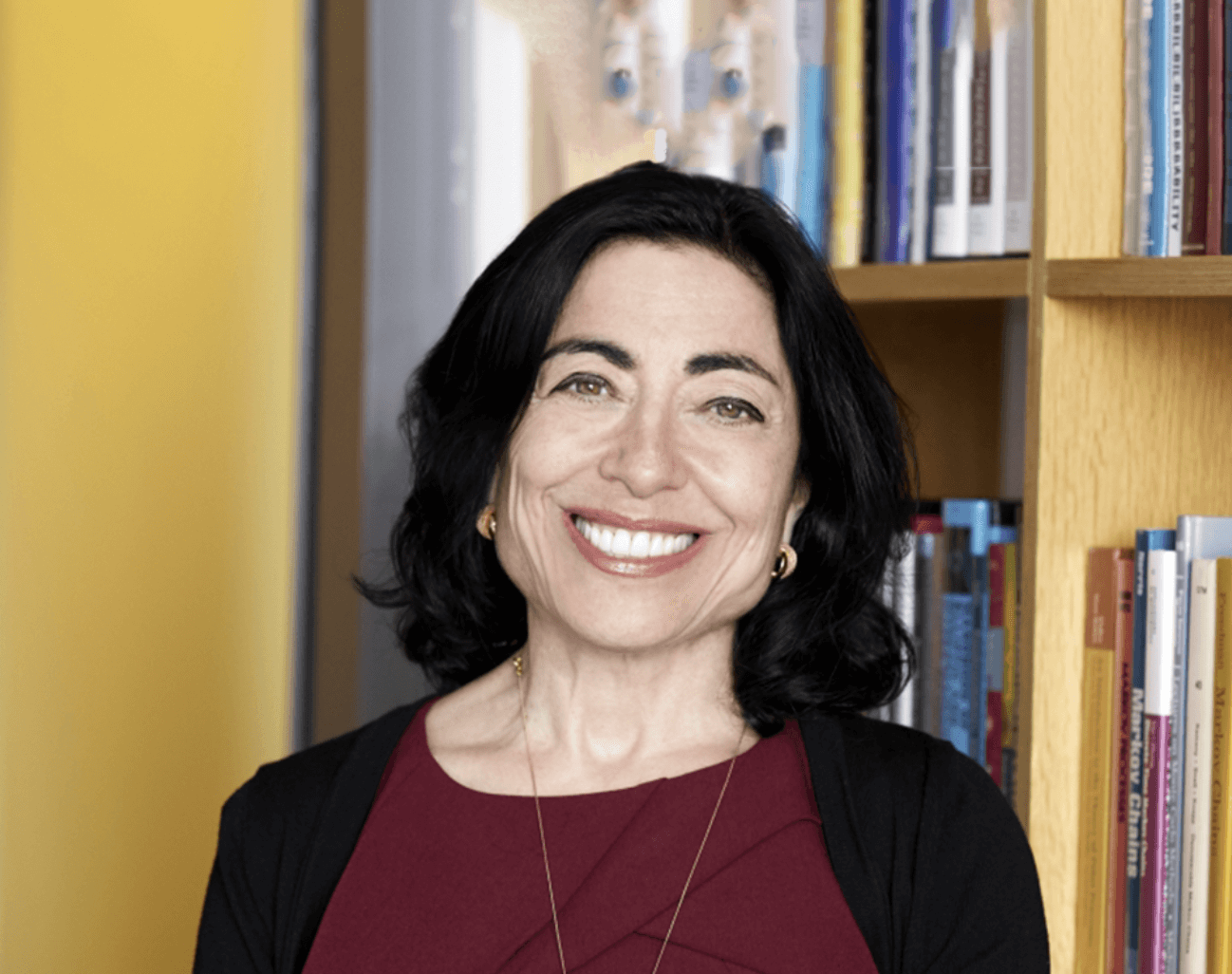
Jennifer Chayes
Jennifer Chayes is Dean of the College of Computing, Data Science, and Society. She is a Professor of Electrical Engineering and Computer Sciences, Mathematics, Statistics, and Information. Before joining Berkeley, she was a Technical Fellow at Microsoft, where she led research programs for over 20 years and was founder and managing director of three interdisciplinary labs: Microsoft Research New England, New York City, and Montreal.
Before joining Microsoft, Chayes was a Professor of Mathematics at UCLA, where she received the UCLA Distinguished Teaching Award. During her time at Microsoft, she was an Affiliate Professor of Mathematics and Physics at the University of Washington. She is an author of about 150 scientific papers and the inventor on more than 25 patents. She earned her Ph.D. in mathematical physics at Princeton University and has been awarded honorary doctorates from Bard College in New York and Leiden University in the Netherlands.
Chayes has received numerous awards for both leadership and scientific contributions, including the Anita Borg Institute Women of Vision Leadership Award, the John von Neumann Award of the Society for Industrial and Applied Mathematics. She is a member of the American Academy of Arts and Sciences and the National Academy of Sciences and a Fellow of the American Association for the Advancement of Science, the Association for Computing Machinery, and the American Mathematical Society.
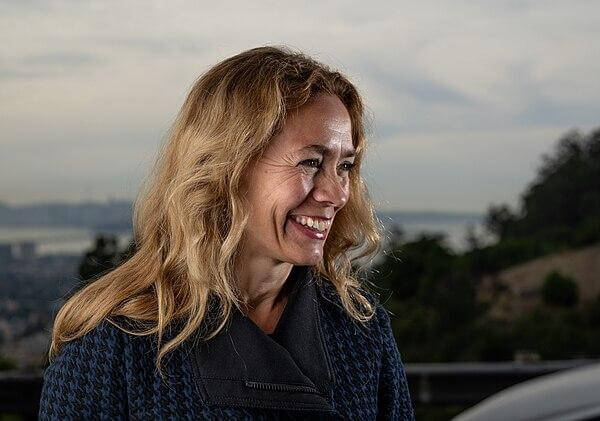
Kristin Persson
Kristin Persson directs the Materials Project which is a multi-institution, multi-national effort to compute the properties of all inorganic materials and provide the data and associated analysis algorithms to researchers free of charge. The ultimate goal of the initiative is to drastically reduce the time needed to invent new materials by focusing costly and time-consuming experiments on compounds that show the most promise computationally. The Persson group uses their expertise in materials informatics and the high-throughput infrastructure of the Materials Project to design novel materials for energy applications and to guide synthesis and characterization through data-driven methodologies.
|
Organizing Committee
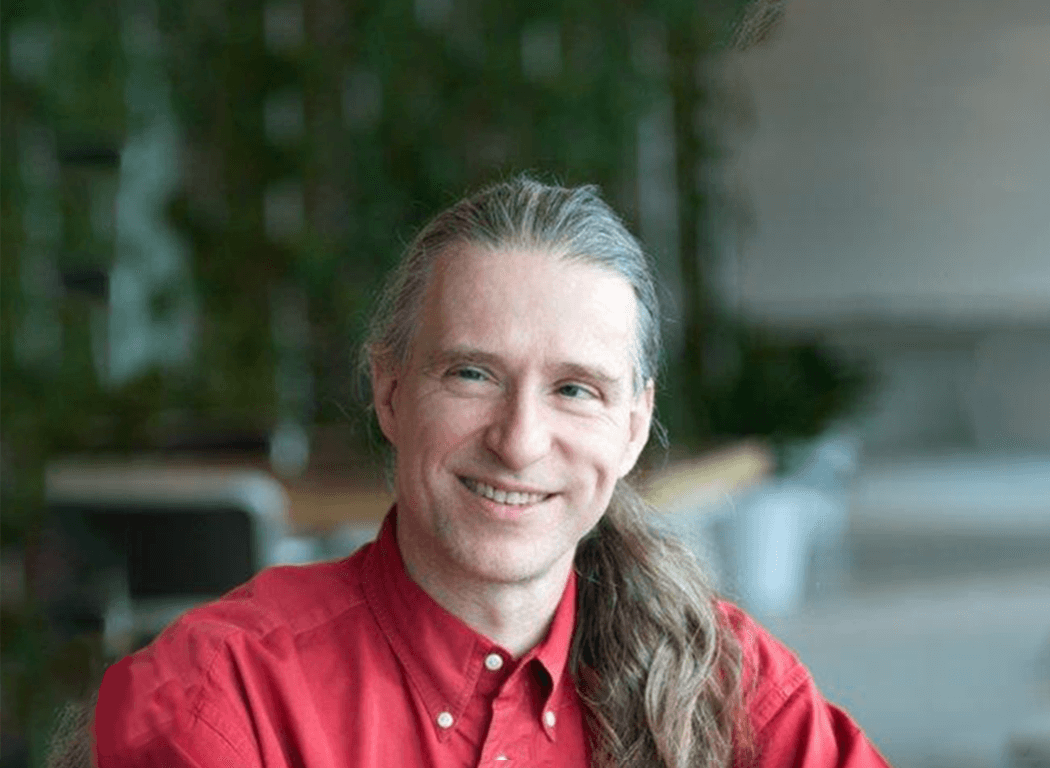
Christian Borgs
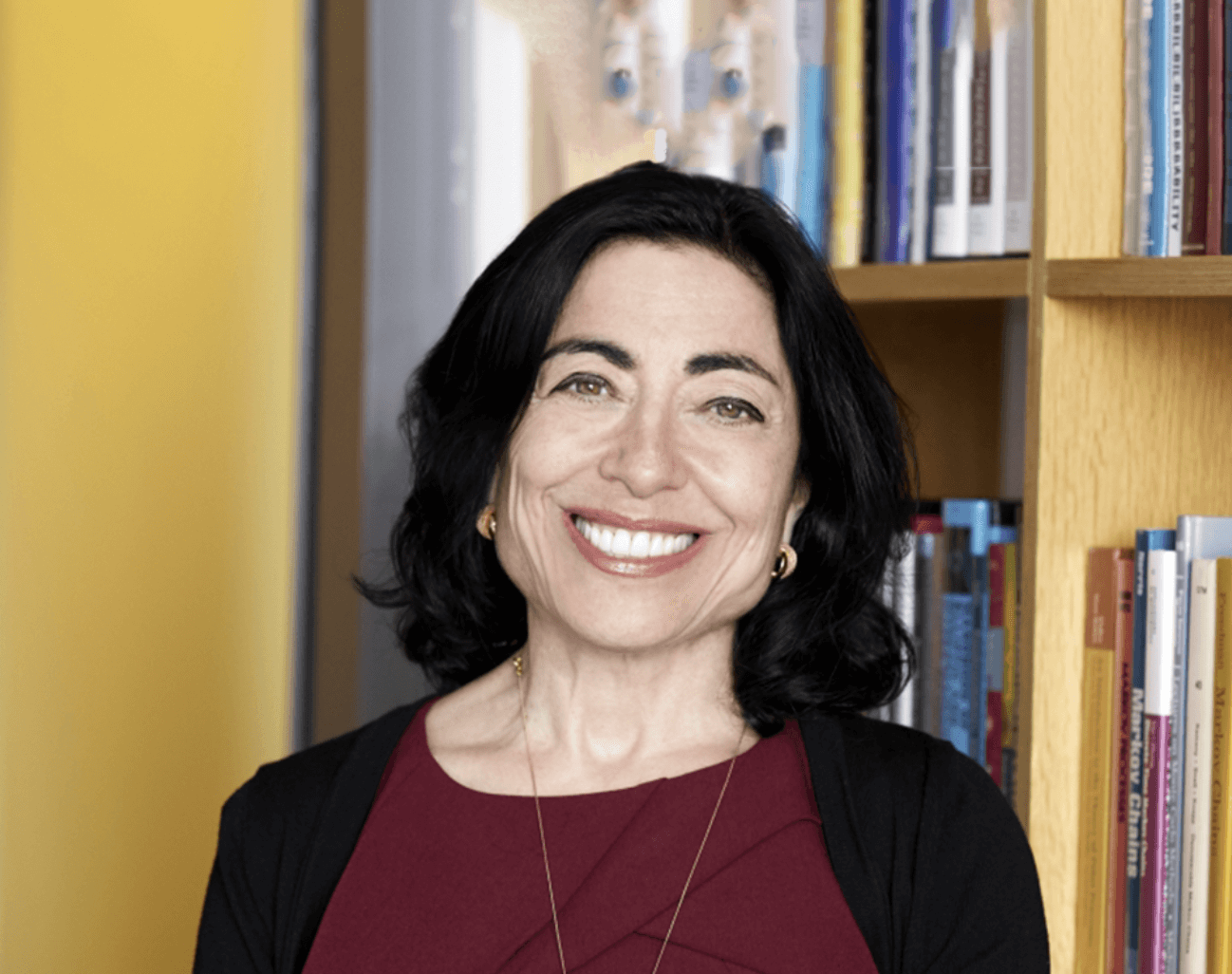
Jennifer Chayes
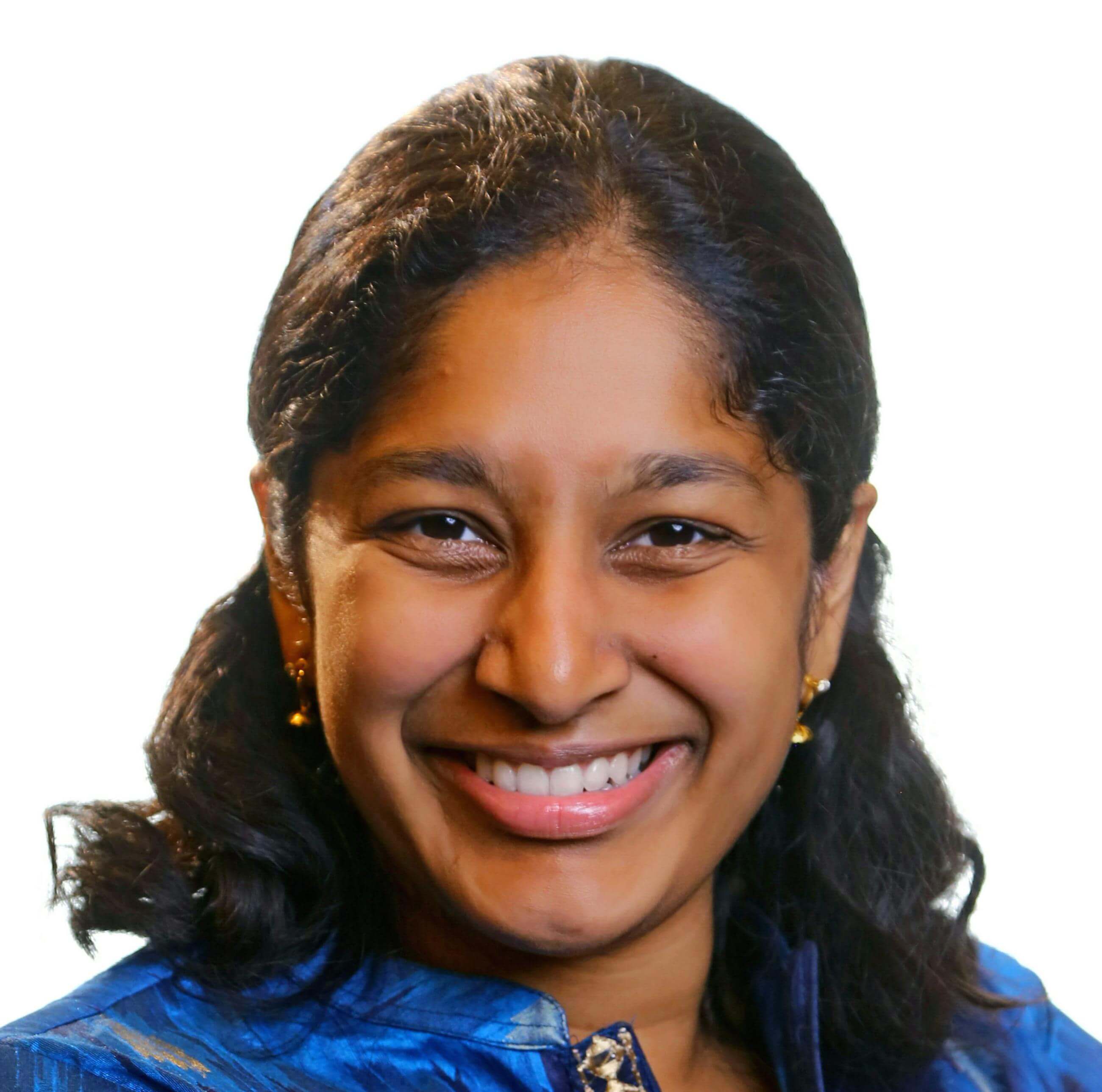
Priya Donti
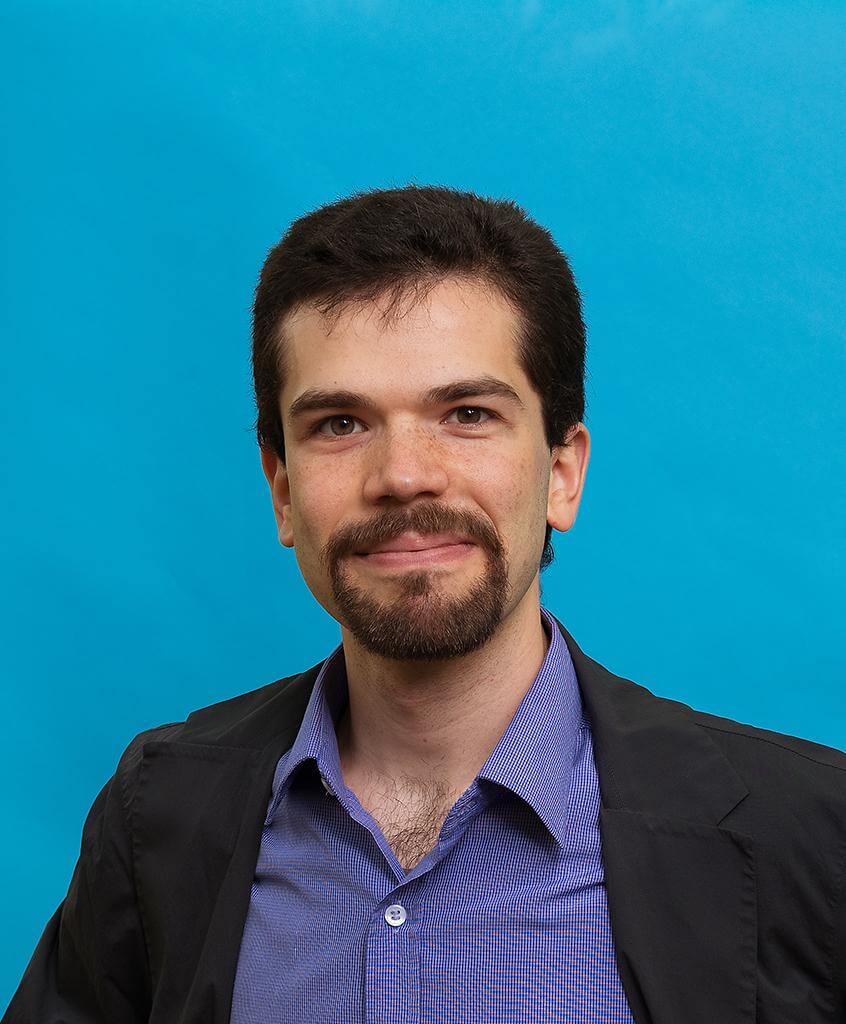
David Rolnick
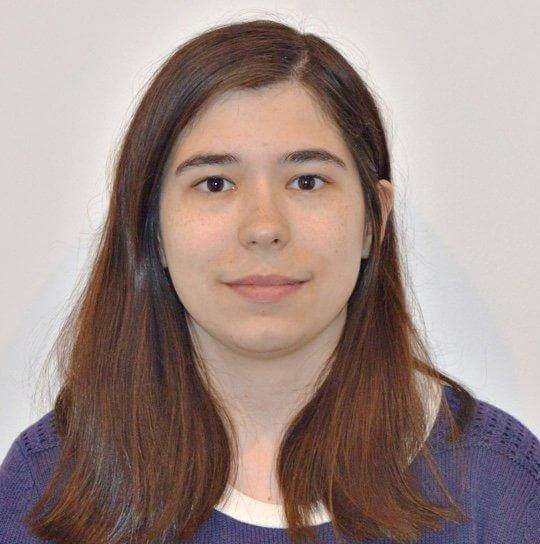